In today's world, where data drives decision-making, Data Quality (DQ) has emerged as a fundamental pillar of organizational success. Companies rely on precise, dependable, and timely data to strategize effectively, streamline operations, and foster growth. Despite its importance, many organizations still grapple with data quality challenges that hinder performance and tarnish reputations.
Data quality extends far beyond technical concerns; it is a strategic necessity impacting every aspect of an organization. Inadequate data quality can lead to costly mistakes, lost opportunities, and strained customer relationships. Conversely, high-quality data empowers better decision-making, enhances operational efficiency, and builds competitive advantages.
This detailed guide delves into the concept of DQ, its significance, associated challenges, and practical strategies for improvement. Whether you're a business leader, data specialist, or anyone interested in leveraging data for success, this article equips you with actionable insights and expert advice.
Read also:Exploring The Rising Star Madelyn Clines Journey Through Hollywood
Table of Contents
- Understanding Data Quality (DQ)
- Why Data Quality Is Essential
- Key Dimensions of Data Quality
- Common Challenges in Data Quality Management
- Advantages of High-Quality Data
- Assessing Data Quality
- Strategies to Enhance Data Quality
- Technologies Supporting Data Quality
- Data Quality in Various Industries
- The Future Landscape of Data Quality
- Final Thoughts
Understanding Data Quality (DQ)
Data Quality (DQ) refers to the overall suitability of data for its intended purpose. It evaluates the accuracy, completeness, consistency, timeliness, and relevance of data within an organization. High-quality data ensures businesses can depend on their information for informed decision-making, process optimization, and goal achievement.
The concept of DQ goes beyond mere accuracy. It encompasses the entire lifecycle of data, from collection and storage to analysis and utilization. Organizations must ensure their data is clean, consistent, and updated regularly to avoid inaccuracies, inefficiencies, and costly errors. By prioritizing DQ, businesses lay a strong foundation for sustainable growth and success.
Why Data Quality Is Essential
In the rapidly evolving business landscape, the importance of data quality cannot be overstated. Poor data quality can lead to severe consequences, including financial losses, operational inefficiencies, and damaged customer relationships. Conversely, high-quality data offers numerous benefits, such as improved decision-making, enhanced customer experiences, and increased competitiveness.
For instance, a retail company with precise customer data can tailor marketing campaigns, optimize inventory management, and elevate customer service. In contrast, a company with subpar data quality may struggle to understand its customers, resulting in missed sales opportunities and customer dissatisfaction. Investing in DQ is, therefore, a strategic move for long-term success.
Key Dimensions of Data Quality
Data quality can be assessed based on several critical dimensions, each playing a pivotal role in ensuring data reliability and usability. These dimensions include:
- Accuracy: The extent to which data reflects the true state of affairs.
- Completeness: The degree to which all necessary data is present.
- Consistency: The uniformity of data across different systems and sources.
- Timeliness: The availability of data when it is required.
- Relevance: The alignment of data with organizational needs.
By focusing on these dimensions, organizations can identify and address data quality issues more effectively, ensuring their data remains a valuable asset.
Read also:The Arrival Of Spring A Season Of Renewal And Growth
Common Challenges in Data Quality Management
Achieving high data quality is not without its hurdles. Some of the most prevalent challenges include:
- Data Silos: Different departments or systems may store data separately, leading to inconsistencies and redundancies.
- Poor Data Governance: The absence of clear policies and processes for data management can result in inaccuracies and errors.
- Human Error: Manual data entry and other human factors can introduce inaccuracies and inconsistencies.
- Changing Data Requirements: As business needs evolve, data requirements may shift, necessitating continuous adjustments to data quality processes.
Overcoming these challenges requires a comprehensive approach involving technology, processes, and people. By addressing these obstacles head-on, organizations can enhance their data quality and unlock its full potential.
Advantages of High-Quality Data
Investing in data quality can yield substantial benefits for organizations, including:
Improved Decision-Making: High-quality data provides a reliable foundation for informed decision-making, minimizing the risk of errors and enhancing outcomes.
Enhanced Operational Efficiency: By eliminating inaccuracies and inconsistencies, organizations can optimize processes, reduce costs, and boost productivity.
Stronger Customer Relationships: Accurate and up-to-date customer data enables businesses to offer personalized experiences, build trust, and foster loyalty.
Assessing Data Quality
Evaluating data quality involves comparing data against established standards and metrics. This process typically includes:
- Data Profiling: Analyzing data to uncover patterns, anomalies, and potential issues.
- Data Auditing: Conducting regular reviews to ensure compliance with data quality standards.
- Performance Metrics: Monitoring key performance indicators (KPIs) related to data quality, such as error rates and data completeness.
Establishing a robust measurement framework allows organizations to track and improve their data quality over time, ensuring it remains a valuable asset.
Strategies to Enhance Data Quality
Implementing Robust Data Governance
Data governance is a cornerstone of data quality management. It involves creating policies, processes, and roles to ensure the effective management and use of data. Effective data governance helps organizations:
- Define clear data standards and policies.
- Assign accountability for data quality.
- Promote collaboration across departments and systems.
Leveraging Advanced Data Quality Tools
Technology plays a crucial role in enhancing data quality. Data quality tools can automate tasks such as data cleansing, validation, and enrichment, reducing the risk of errors and inconsistencies. Some popular data quality tools include:
- SAS Data Quality
- Informatica Data Quality
- Talend Data Quality
These tools enable organizations to streamline their data quality processes and achieve better results, ensuring their data remains accurate and reliable.
Technologies Supporting Data Quality
Beyond dedicated data quality tools, emerging technologies are revolutionizing how organizations improve their data quality. These include:
- Artificial Intelligence (AI): AI-powered tools can analyze data patterns and automatically identify potential issues.
- Machine Learning (ML): ML algorithms can predict data quality issues and recommend corrective actions.
- Blockchain: Blockchain technology enhances data integrity and traceability, ensuring data accuracy and consistency.
By embracing these technologies, organizations can elevate their data quality efforts and stay ahead in an increasingly data-driven world.
Data Quality in Various Industries
Data quality is relevant across all industries, though the specific challenges and solutions may vary depending on the sector. For example:
- Healthcare: Accurate patient data is vital for delivering safe and effective care.
- Finance: High-quality financial data is crucial for compliance, risk management, and decision-making.
- Retail: Customer data quality impacts marketing effectiveness and customer satisfaction.
Each industry must customize its data quality strategies to address its unique needs and challenges, ensuring data remains a strategic asset.
The Future Landscape of Data Quality
As data continues to grow in volume and complexity, the importance of data quality will only increase. Emerging trends such as big data, IoT, and AI present new opportunities and challenges for organizations striving to enhance their data quality.
To remain competitive, businesses must adopt a proactive approach to data quality management, leveraging technology, expertise, and collaboration to ensure the accuracy, reliability, and usability of their data. By staying ahead of the curve, organizations can harness the full potential of their data for sustained success.
Final Thoughts
Data Quality (DQ) is a critical element in achieving business success in today's data-centric world. By recognizing the significance of data quality, tackling common challenges, and implementing effective strategies, organizations can unlock the full potential of their data. We encourage you to evaluate your current data quality practices, identify areas for improvement, and adopt the strategies outlined in this guide. Your feedback and insights are invaluable, so feel free to share your thoughts or this article with others who may benefit from it. Together, let's shape the future of data quality and drive meaningful progress.
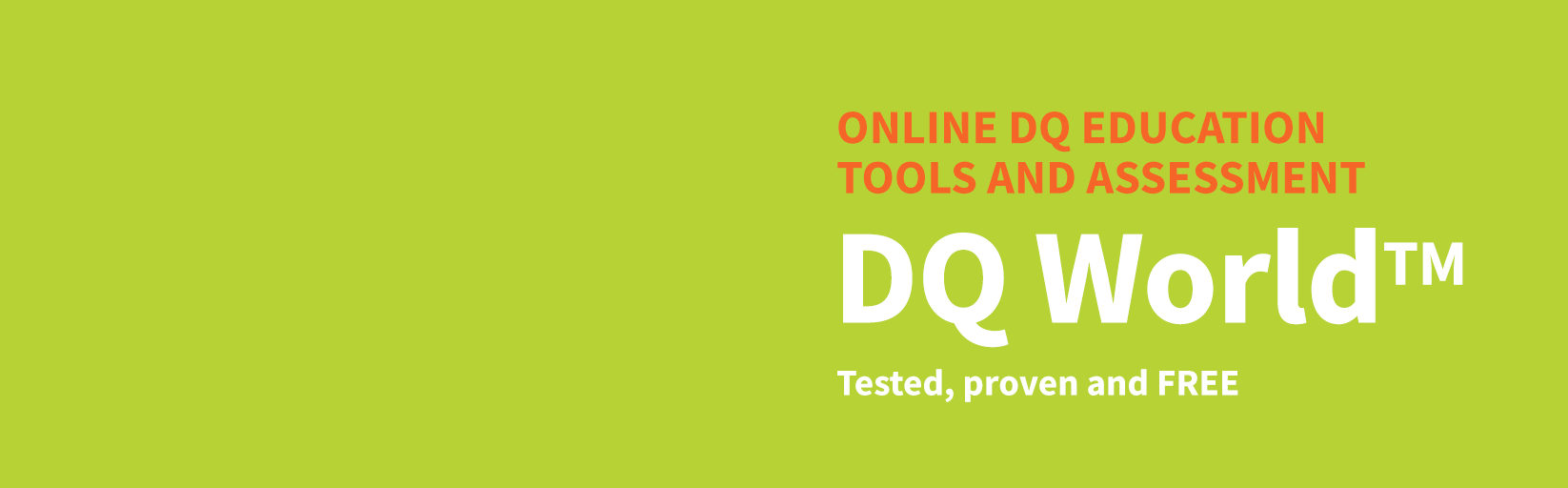
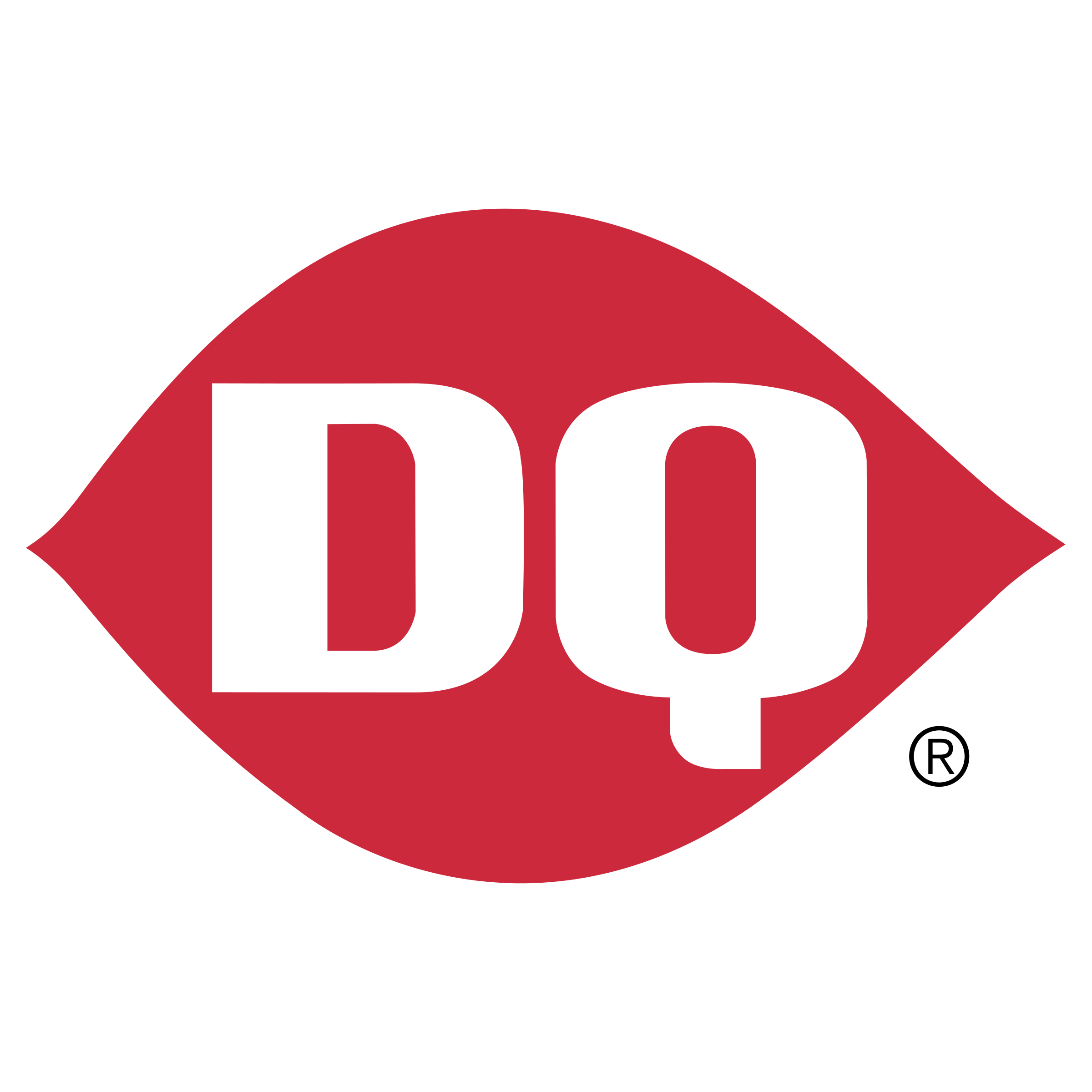
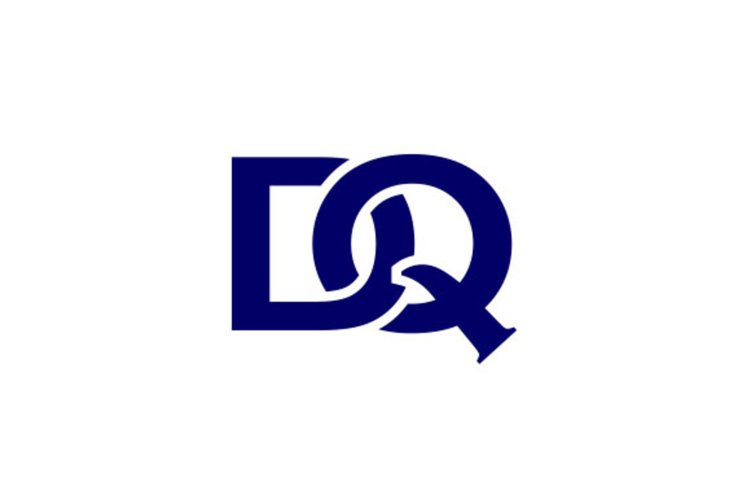